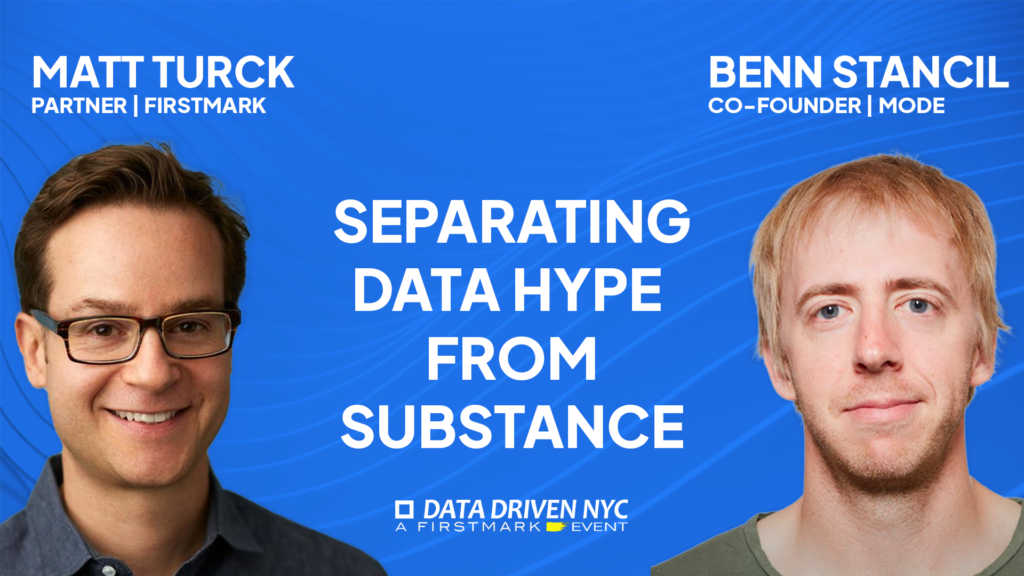
In addition to his role as co-founder and Chief Analytics Officer of Mode, a leading collaborative data platform, Benn Stancil is a prolific and thought-provoking writer about the broad data space. Over the last couple of years in particular, he’s produced a series of insightful and entertaining posts on his newsletter: https://benn.substack.com/
We had welcomed Benn at Data Driven NYC back in 2019 to talk about Mode (see the video, “The case for hiring more data analysts“), and it was great to have him back from a wide-encompassing conversation where he addressed some of the “sacred cows” of the data world.
One of the most interesting conversations on the space we’ve had recently, highly recommended watch!
Video and transcript below
As always, Data Driven NYC is a team effort – many thanks to Katie Mills, Drew Simmons, Dan Kozikowski and Diego Guiterrez for all the work and support.
TRANSCRIPT:
Matt Turck (00:12):
Benn, welcome back. You spoke at the event in 2019, which feels a decade ago.
Benn Stancil (00:19):
15 years ago. Thanks for having me.
Matt Turck (00:21):
But actually, not that long ago. So, you are the Co-Founder and Chief Analytics Officer of Mode, which is a collaborative platform for data analyst and data scientist.
Benn Stancil (00:33):
Yeah, correct. So, I’m one of the founders of Mode. We started it just over nine years ago, so it’s now been a while. It is a BI tool basically, but a BI tool built for people who don’t like BI. So, it’s like-
Matt Turck (00:46):
Conflicted people.
Benn Stancil (00:47):
Yeah, exactly. That are analysts that have to provide BI but don’t really want to do it. And so, I do a few different things there. My title is technically Chief Analytics Officer. It’s a made-up title because when you start a company, you can make up a title.
Matt Turck (00:58):
In fact, that’s why you start a company.
Benn Stancil (01:01):
Yeah, exactly. It’s all for the LinkedIn. So, my job there is twofold. It’s a lot of, basically, talking to folks in the community, trying to figure out where the space is going, where Mode wants to be. And then, a lot of products work, funneling that back into the things we build, the way we talk about it, what we can do to provide things for our customer, stuff like that.
Matt Turck (01:20):
Okay, very cool. And one major thing that has changed since we spoke in 2019, at least, I believe, that you started a blog or Substack, which I personally love. And look, I don’t say that about everyone. I think Benn’s writing is super brilliant and provocative and interesting. So, I’ll do the plug so you don’t have to do it. So, it’s Benn, B-E-N-N .substack.com?
Benn Stancil (01:49):
Correct.
Matt Turck (01:49):
And you write very prolifically every week. So, it is actually a great place to start for a lot of people who are in technical roles or product roles in technical companies. There’s been this rise of people writing interesting content but professional content. So, why do you write?
Benn Stancil (02:14):
So, when we first started Mode, it was three of us. Our CEO who was presentable and could talk to investors and customers. The guy who was our technical co-founder who was our CTO, who was actually building the product. And me, who was neither of those things and had no real job.
(02:30):
And so, back then, what I did was I wrote a blog and it was a blog that was… we had no product and nothing to advertise. So, it was basically a blog about data adjacent things that was… it was like pre-538, but it was 538-ish stuff. The very first blog on Modes corporate blog is a post I did three days after we started the company that was about Miley Cyrus and the VMAs.
(02:55):
And so, I did that for six months because I had no other job. Ad it actually worked reasonably well as like, okay, this got some data people interested in what Mode was. They had no idea what the product was. It was like these people are talking about stuff that seems interesting, even if it’s not terribly relevant to what I do day-to-day.
(03:12):
Over the course of my time at Mode, you bounce on a bunch of different jobs. You did stuff in support and product and marketing and solutions and all these different things. At some point, basically, everybody at Mode realized I’m not good at any of those jobs and I slowly got myself fired from all of them.
(03:27):
And so, I’m on my way back to doing a blog, this was about 18 months ago, I started doing it with the intent of it being back to that original, just right about data related things. It took on a life of its own of like, well, I’ll figure out stuff that’s interesting that evolved a lot into what’s going on in the data world, because a lot things have changed from what it was in 2013 to now.
(03:49):
And so, it ended up just falling into this habit of, all right, do it once a week. Talk about commentary on the data world, I guess. It doesn’t really have much of an editorial direction, but I don’t know. At this point, I do it for my entertainment and just trying to stay on top of what’s going on. And I don’t know, to think out loud in a lot of ways.
Matt Turck (04:10):
And for anyone that’s in startups and thinking about content marketing and technical writing and all those things, beyond your own entertainment, do you try to trace this back to any metrics or lead generation or any of those things? I mean, I can certainly vouch for the fact that everybody in the data world reads this thing, so it’s usually influential. But do you have a metrics attach to it?
Benn Stancil (04:33):
Much to our marketing team chagrin, we do not. So, Substack doesn’t do a great job of helping you out here. We have metrics of like I follow how many people subscribe to it and you can look at traffic to it. And it goes up on Fridays and goes down on Saturdays.
(04:49):
In terms of tying it back to driving leads at Mode, not really. And in a lot of ways that’s not the goal. I started doing it as a let’s see what happens. Now, there is some push from, as would make sense, from folks in the marketing team and stuff to be like, all right, what do we… we need to actually deliver some value here.
(05:11):
And so, a lot of though I think is, to me, the value of it is it’s not marketing content, it’s not going to be at the end of it. And by the way, Mode solves this problem, buy Mode. I don’t want it to be that. That doesn’t mean there aren’t ways to turn it into something that’s useful or turn the brand into something useful or whatever.
(05:29):
But that’s a little bit of a work in progress to us. And to me, it was like, all right, write it. Do it for something that’s interesting and fun and see what happens. And then, if it works, figure it out from there. If it doesn’t work, I guess, I’ll yell at my quarter on the internet and never pay attention.
Matt Turck (05:44):
Okay, great. So, there’s so many gems in that, but I’d love to dig into some of them. One that I personally think a lot about is the 10,000-thousand-foot view, market overview if you want, of the modern data stack, which is called-
Benn Stancil (06:04):
The 10, really?
Matt Turck (06:06):
No, pontificate endlessly. It’s living. And you called it both a powder keg and a Ponzi scheme, and I’d love to go into that. And maybe to make this super interesting and relevant for everyone, just start it with a quick definition of what actually the modern data stack means, which is not always what people think it is.
Benn Stancil (06:31):
So, my definition of the modern data stack, to me, it’s data companies that launched on Product Hunt, it’s like an imprecise definition. But to me, the question, so modern data stack generally I think is modern data tools, has modern architecture, it’s cloud-based.
(06:50):
It’s meant for analytics teams and not traditional BI developer teams. How exactly you draw lines around that people can debate. My view of it is it’s basically products that are meant to sell in a bottoms up motion. The Product Hunt thing works because one, it ties to the timing, that’s roughly when things started.
(07:08):
When Product Hunt became a thing, it’s roughly when all these tools started coming out, the early ones like Looker and FiveTran and all those things. One of the questions I have when people ask like, what’s the modern data stack is Oracle released a new cloud data warehouse, is that a part of the modern data stack? And if it’s like no, it’s going to… why not? You’re just hating on Oracle.
Matt Turck (07:28):
It’s not cool.
Benn Stancil (07:29):
Yeah, it’s just not cool enough, I guess. I suspect that wasn’t on Product Hunt, I don’t know. I don’t know if Product Hunt’s cool anymore or not either. But anyway, that fits the brand to me. So, I think it’s all of the tools in that space that a lot of things are for data practitioners, a lot of them are for data adjacent people.
(07:48):
A lot of them are data tools that are being brought to marketers, to product people, to engineers. But basically, anything you can put on your diagram to me roughly fits into that category.
Matt Turck (07:57):
So, why is it a Ponzi scheme then?
Benn Stancil (08:03):
It’s a lot of companies-
Matt Turck (08:04):
First, this is not a crypto conference, but we do talk about Ponzi schemes as well.
Benn Stancil (08:08):
Actual Ponzi schemes. So, the problem to me is there’s too many companies basically selling two smaller problems that it’s still expensive to build a data company. We don’t yet have the iPhone appification yet of data products where you can build an iPhone app with a couple people.
(08:30):
It’s pretty cheap to build. If it takes off, great, you can turn it into something bigger. But Instagram was 50 people when it was worth a billion dollars. WhatsApp was like 10 and everybody became billionaires. All these companies could get really big because the platform is there to support, being able to build a very rich application without a whole lot of investment.
(08:50):
And so, you can have thousands and thousands of apps because the market can support them, and the market can support ones that don’t make a whole lot of money. The data world still is like it’s pretty expensive to build a data product. You got to go out, you got to go raise venture money.
(09:02):
If you’re raising venture money, you’re going to expect to have a pretty bigger return and you’re going to expect to have make a bunch of money. All those companies are chasing and their pitch decks are chasing, here’s our path to a hundred million dollars.
(09:13):
Market is big, it ain’t that big. And what ends up happening, I think, is a lot of these companies are chasing these fairly narrow wedges that feel big in the moment when everybody’s excited about it, but pretty quickly they’re going to realize they’re all stepping on each other’s toes and that fallout has to go somewhere. Not all of these companies can be the next Figma that they all now say that they are.
(09:37):
And so, it’s what happens then. And I think it’s somewhat of a reckoning has to come. There may be some softer landings and stuff for folks in ways out, but it seems very difficult for these companies. The slide you create does not have a thousand-billion-dollar companies on it. It’s just like that’s a trillion-dollar market and no. It’s popular, it’s not that popular.
Matt Turck (10:00):
And you were saying in the last couple of years in particular throughout the VC environment, there was a little bit of data people in companies that actually knew where they were talking about, left their companies to start a company. And because all the data people left, the companies had to buy the product that those people left built?
Benn Stancil (10:19):
Yeah. So, to me, this all peaked in this. There was a conference in Austin, it’s called Data Council. Good Conference, ProCon for that conference, no striking to that conference. The timing of it was just too perfect where it was this… the first big in-person data conference among the modern data stack community.
(10:39):
It was this big celebration of the modern data stack. Airflow acquired, I mean, not Airflow. Astronomer acquired a company in the middle of it. It was also right as the market was teetering. And there was this moment of, I don’t know, like dancing on the deck of the Titanic a little bit of, wait a minute, this doesn’t… is this going to… are we going to have this party next year?
(10:59):
Because I don’t know if we’re going to have this party next year. But anyway, in response to that conference, a couple people were saying basically there are a lot of data practitioners there who become founders, and they viewed it as these people are inevitably going to be successful.
(11:11):
Because when data practitioners start companies, they create more of a market for more data people to sell to. And there are fewer data people to be able to build data products internally, so we have to go buy them. And it’s like how can this all fail? And it felt a little bit like how our housing price is going to go down in 2007.
(11:27):
And so, it doesn’t seem like it’s going to really hold up. I think there will be a lot of money made, a lot of really good companies built, but it’s in the very explosive, expansive phase to me where there’s a lot of people chasing very narrow wedges that when push comes to shove, they’re going to have to be like, oh, we actually need to be a much bigger product to be able to make a path to a hundred million dollars.
Matt Turck (11:49):
And in various blog posts you go with a lot of vigor and enthusiasm after some of the industry’s sacred cows. So, one by one and maybe starting with Snowflake, which is the company everybody loves, and that’s actually the most highly valued software company in the world in terms of multiple.
(12:12):
And you wrote very interestingly, which I think is a fantastic thought exercise. You wrote a bug post about the scenarios where Snowflake would actually fail. Just walk us through the thesis.
Benn Stancil (12:27):
So, I’m bullish on Snowflake. I don’t think Snowflake’s going to fail. They seem to be smart. They seem to be doing well. But it is them along with a few other folks have become this default where we assume, okay, Snowflake is going to take over like Larry Ellison’s going to be dead, we’re all going to use Snowflake.
(12:47):
Oracle is gone. It’s going to be the next trillion-dollar thing. And to me, the interesting question there is, okay, let’s assume it’s not. Let’s just assume in five years something has gone horribly wrong because there is a path to somewhere. So, there’s some timeline on which that is where we end up.
(13:02):
How about we get there? What does that actually look like? And the current set of thinking around Snowflake is, well, it’s expensive, that data tools are extremely indiscriminate in the amount of load that they put on Snowflake. One of the nice things about Astronomer is anybody could run queries at Snowflake.
(13:21):
You know who really loves that? Snowflake. Who doesn’t love it? The people who pay the bills for Snowflake. And at some point, that becomes problematic. But I don’t think that, to me, that doesn’t really represent a real threat because that’s basically, Snowflake died because it was too popular.
(13:37):
It’s like, well, okay, they’ll probably figure that one out. I think the more interesting question for Snowflake is at their conference in the summer, they released a ton of new features. It’s no longer a database. It’s like this whole platform that is… it’s an app, like a layer for building apps.
(13:56):
It’s a bunch of other data management tools. They want to build more things on top of it. It can be a transactional database potentially. There’s a question to me whether or not those bells and whistles stick. And if they don’t, what I feel like you end up with is an extremely complicated and overpriced database that you just want something that has horsepower.
(14:15):
So, I remember a couple years ago, this was now, well, this was eight years ago, pandemic. I was trying to buy a TV. And I just wanted a TV that played videos. And you go into Best Buy and they have a bunch of smart TVs. And it’s like, oh, this one can turn on your dishwasher.
(14:35):
And I’m like, I don’t… it doesn’t make sense but okay. And so, I ended up finding a TV that was just a TV. And to me, it’s like the question is does the market want a database that can turn on your dishwasher? That is all of these other things, that is this giant data platform that will cost a lot but is okay because it has all these features.
(14:52):
Or, does it want just something that is performant and is a TV? And there’s a lot of new technology of things like DuckDB and stuff like that, that if you just want a TV, that might be better. And then, you can run that TV on bare metal AWS. You can run it for way less price than you’re probably paying for Snowflake.
(15:10):
So, I think that’s the real question, to me, is if Snowflake can make all of these things one single package where you can’t buy the TV without the other pieces like that is… the database is all of these things now. I think they’re in a really good spot.
(15:23):
If they can’t and it feels like I’m adding a bunch of add-ons I don’t actually want, then I think they’re still probably will be fine but you run the risk of getting really undercut by someone who just says, “I will sell this thing to you at cost” basically, that they can probably perform more or less the same way.
Matt Turck (15:39):
And even if they want to be all those things, they’re going to be competing for different features with different people like the Fireball to for interactive queries and Databricks and a bunch of others.
Benn Stancil (15:52):
And there’s another version of this that goes even in the more extreme direction of maybe we don’t want just a TV, maybe we don’t just buy a house in a box. Where if Google figured it out, Google, to me, is one of those companies that’s like, what are you doing?
(16:07):
They have a ton of technology to be able to solve all these problems, and they really buy an entire data stack in one fell swoop. They haven’t pieced it together yet. But I think that’s another place where something Snowflake comes a little bit under risk if we start to buy data products the same way we buy cloud on infrastructure.
(16:25):
Where if you’re using GCP, chances are you’re just going to use GCP for everything. You may be multi-cloud but you’re not going to buy one GCP service over here and one AWS service over here and Azure over here. You’re going to buy them all to work together. I could see the data world moving in that direction because there’s so much… the ecosystem is so big.
(16:44):
Fine, AWS has a dropdown of 300 services. Chances are, I’ll just choose the one from them. Then Snowflake is trying to compete with the packaging of Microsoft, of AWS, of Google. And that’s a little bit of a tougher compete too, but I think that’s probably not the direction it goes.
Matt Turck (17:02):
So, that’s Snowflake. Let’s talk about FiveTran and ETL and maybe just in one minute. What is FiveTran and what is ETL? We had George Fraser, the CEO at this event online during the pandemic, but maybe as a refresher.
Benn Stancil (17:19):
So, FiveTran is the far left of this diagram you all just saw. You got a bunch of data in third-party sources or in data warehouses. You want to centralize it into your central warehouse, be at Snowflake or Databricks or BigQuery or whatever. The way you had to do that before, the first data team I worked on in Silicon Valley did this, you had to basically write a bunch of stuff to scrape things out of APIs of these services.
(17:43):
So, you’d have to basically hire an engineer to scrape stuff out of Salesforce’s API. It was an enormous pain. The API is actually decent but it’s still like you have to manage it. When things change, you have to fix it. FiveTran does it all for you. So, FiveTran is basically pull data out of various services.
(17:58):
They connect to a couple hundred now, I don’t know how many… you push a button, you say sync the data from the service into your warehouse and they just do all of it for you. So, it’s essentially a copy it from thing that doesn’t quite look like a database into a database, and then you can build all the stuff you just saw on top of it.
Matt Turck (18:16):
And it’s companies that’s been around for about 10 years and it’s actually, as far as I know, one of those companies are over a hundred million in revenue. So, what’s the case against, not necessarily them, but that space?
Benn Stancil (18:28):
So, to me, the potential question there is, it’s a little bit of an awkward thing for a company to be sitting as this middleman. What they essentially do is they sit in between… take Salesforce and Snowflake. They sit in between those two. They have to maintain a connection to Salesforce’s APIs.
(18:47):
When Salesforce changes it, which Salesforce doesn’t care what FiveTran does. I mean, FiveTran is may be big enough now that they do a little bit, but third-party services aren’t going to go call FiveTran and be like, “Hey, we’re changing our API, fix it.” So, FiveTran basically has to maintain that.
(19:01):
The way they also get data out of it is they scrape it. Some companies provide ways for like we are making changes, they push it to other services. But a lot of times, it’s just run a script against the API, check the differences and put the thing back into the database and batch.
(19:18):
There’s a clunky way to do this. It would be more sensible if you could design this in a perfect world that Salesforce just writes it to a database. Now, obviously, they didn’t do that way back when because nobody wanted it. But now, it’s become such a thing to say, “Hey, we want our database. Our data out of your SaaS software into a database.”
(19:34):
Not for the sake of migrating away from Salesforce, but for the sake of all the analytics that we’re going to go on top of it. Salesforce could just provide that directly and say, “Okay. We’ll connect to Snowflake.” They actually just released a partnership that’s dancing in this direction a little bit.
(19:48):
But SaaS services could do this where they just write essentially directly to databases and they basically take the cut that FiveTran is paying. So, instead of me as a data team saying, “I’m not going to go buy FiveTran to do this, I’m going to pay them 10K a year to sync data from A to B. I’ll pay 8K to the SaaS service to do it.”
(20:07):
They’ll probably do a better job because they’re maintaining the SaaS service already, they know when it changes. They can push rather than pull. And so, it’s a little bit of a better setup. It just makes more sense.
Matt Turck (20:19):
Have you seen people starting to do that?
Benn Stancil (20:22):
So, there are some companies that have done this before. Companies like Segment, basically, Event Tracking Services did this because that’s the product. Stripe has a way to do this. There’s a few that have some crude versions of this. I actually talked to George a little bit after that post.
(20:44):
His take is, which I think is probably fair, is it’s a lot harder to build that than you think. That the reason FiveTran is a $6 billion company or whatever is because they did a bunch of awful work that none of us want to do. And so, as a SaaS business, Mode could do this.
(21:00):
Mode could build a thing that syncs stuff to Snowflake. We’re not going to because we have other things to build. And sure, we could monetize it but it’s not really worth it. We’re not looking for something marginally makes us more money. We need to make things that are going to make us 10x more money.
(21:13):
So, I think that’s the reason we don’t. The one thing to me that changes that dynamic is if Snowflake or Databricks or whoever start to say, “Hey, we want to make it really easy for people to be able to do this.” And we build services that make it so that we can, in a week, build that connection to Snowflake so they have an app layer essentially.
(21:32):
But instead of it being something built on top of Snowflake, it’s more of an ingestion app layer, where we can just write to that thing and Snowflake handles all the complexity and it’s like, okay, we would do that. And then, we would go off and sell it and stick in an enterprise tier, because you’re always chasing features to put in an enterprise tier.
(21:46):
So, I think that’s how you get there. But it doesn’t undercut everything for FiveTran, but it potentially undercuts the big sources, which I imagine are the things that are the real drivers of revenue for them.
Matt Turck (21:59):
And the upcoming one is dbt. And we had the Tristan, the CEO of dbt just a couple events ago. And just again, to rephrase all of this. All of this is done with love and just as a way to think through where our industry is going as opposed to criticizing anyone in particular. But the post on dbt has not come out. Can you give us a little bit of a preview?
Benn Stancil (22:26):
What’s the preview of the DBT one? That it’s fundamentally wrong, basically, that DBTs a transformation tool. They’re moving in the semantic layer tool. So, basically, they’re saying give us raw data and we will tell you, like apply semantics to it.
(22:46):
The way that they do that now is through SQL. So, semantics are air quote semantics. It’s basically semantics as messy data to a clean data set. It’s not really semantics. It’s not really connected together in a real way. It’s not a model. The analogy I’ve used for this before is dbt is, basically, because you create a bunch of tables.
(23:09):
The model is essentially an animated movie where each shot is independent of the other one. They’re connected in a DAG, but they’re not really logically connected. If you want to build a real model, you probably want something from Pixar.
(23:22):
Or, if you want to shoot a different shot, you actually can just say, “Point it from that direction” and it’s going to be the same thing. Whereas in dbt’s case, if you point it from the other direction, you got to make a new model, and that model could be different like you could draw Aladdin with a hat on differently or whatever.
(23:39):
To me, as they move in this semantic direction, move towards things like metrics, move towards things real time computation. It may be that the sequel approach, define it all in queries and tables doesn’t work anymore. Where you’re starting to be like, “Oh, we actually need ways to define joins.”
(23:59):
We need ways to define these relationships. And you start to edge towards like, “Oh, dbt is a bunch of tables with LookML built on top.” But it’s going to be a weird LookML. And then, it’s like I think you potentially get yourself in trouble there because the fundamental framework that dbt is doesn’t quite make sense anymore.
(24:18):
And so, then, you’re rebuilding semantic models that people have been building for 20 years on top of a weird footing and you’re also way behind. And so, I think that’s… dbt is I think really popular because it’s so easy to get up and running, but it may also eventually be like if it had an undoing.
(24:35):
To me, that would be the undoing is the thing that was really easy to get up and running doesn’t actually solve the real problem that we need to solve down the road.
Matt Turck (24:43):
You just mentioned DAGs in passing and you had some really funny analogies with how airports work. Do you want to maybe remind people what a DAG is and why it may or may not make sense in the data world?
Benn Stancil (24:58):
Yeah, okay. So, I mean, the astronomer folks will define this much better than I can, I’ll attempt to do them justice. It’s basically a series of steps where you go A to B to C. Where you’re going in one direction and it’s dominoes where one knocks over the next one.
(25:13):
And it can be very… there’s a very complicated domino things where one domino somehow knocks over 50, and then there’s 50 funnels into one and they come back to each other and they draw a picture of Tupac face. But you have all of these, essentially, these tasks that line up and are sequential to one another in some way.
(25:32):
To me, okay, that makes sense. But if you’re thinking about orchestrating stuff, the thing I care about as a consumer of this, like I’m a pointy haired executive in some ways now is I want a thing delivered at a certain time. I care about when the end product arrives to me.
(25:50):
I don’t actually care about when I knock over the first domino. That all is like, you tell me, you figure that out. The demo was, okay, we need to have this model set up so that an executive gets a thing at 5:00 A.M. when they wake up in the morning and they’re checking their phone before they do whatever.
(26:07):
The thing I care about is that 5:00 A.M. thing, not the various steps that have to happen before. But the way we’ve built DAGs are like, when do I do start this? When do I kick over the first one? And then, we line it up such that we hope the thing arrives at the end.
(26:21):
And the way it would make more sense to me is you just tell the thing. I need this thing to be here by 5:00 A.M. You figure out what has to happen beforehand and then kick over the dominoes when they need to be kicked over. And so, the airport analogy to me is the way you would actually schedule flights in an airport is you decided when the flight’s going to happen.
(26:39):
And then, the airport’s going to be like, okay, we got to take this flight off from New York to San Francisco. Okay, we’re going to have to have certain people to be ready for it, to be doing the bagging for it, to be loading the plane, all those sorts of things.
(26:52):
And eventually, that backs into, well, when are people going to arrive at the airport. When is the train going to get here, all that stuff. What you shouldn’t do is be like, all right, we’re going to have a bunch of taxis arrive at the airport. When a certain number of taxis arrive, then we’ll check people in the gate.
(27:05):
And then, once they’re there, we’ll put them in the plane. And the plane will take off whenever that finishes, and it’s like that doesn’t really make sense. But that’s how we structure these processes, it’s not quite. But to me, it would make a lot more sense if the system could just be, define the end product you want in a declarative way.
(27:22):
And then, if you understand what needs to be orchestrated to do it, okay, you just go do it. I don’t want to know your process. I just want to know my thing is going to be there when I need it to be there.
Matt Turck (27:32):
All right. Maybe one last one from your mini gems. Let’s talk about data products and the data mesh and where, say, we had Jamaica at this event as well. So, we had all those people and who are fantastically smart and interesting folks. But I’m curious about your take and same deal. If you could just describe what it is first and then go into the thesis.
Benn Stancil (27:57):
Nobody has any idea. I cannot describe either of those things because they have no definition. Data products are a few things, maybe. There are data products are sometimes considered data apps. When people say data apps, they usually mean a blinged out dashboard.
(28:21):
It’s a dashboard with some widgets. A data product, I guess, is a data app that can write back to the database and is interactive in some way. All right. I guess, that’s fair. My view in the example I’ve used before on a data product is, I think, Yelp is actually the best example of a data product.
(28:46):
I don’t know how I define that, but it’s a product that solves a problem that is not a data problem, but fundamentally you can’t remove data from it. That ultimately what Yelp is, is serving me a bunch of data, that’s all it really is. It’s like a bunch of tables but presented in a way that allows me to use it to solve exactly the problem I want, which is where do I eat tonight?
(29:10):
Yelp could be a dashboard. It could be a BI tool with some widgets. I mean, as a data person, it would be fun to play around with it and stuff. But generally, it would be a pretty terrible experience to log into Yelp and you get a Looker dashboard. No knock-on Looker, but I don’t know what I do with that.
(29:30):
So, to me, data products are more of what is the product experience from what problem are we solving. How is data incorporated into that? If we can make data a fundamental part of that, then that’s more of a data product. So, it’s a vague thing. And I think that’s where if we think about what does the modern data stack go, I think it’s serving products like that.
(29:54):
Another example, I think, I’ve used before is Figma, worth a bunch of money now. If I’m a designer in Figma, one thing that I might want to be able to see is as I’m designing screens of an existing UI, how much do people actually use those things? What are the experiences that people are actually touching in that UI?
(30:10):
You could potentially incorporate data into that such that the data surface to people in the moment they need it, in the product that you’re trying to use to solve the problem instead of going to a dashboard and clicking on some stuff. So, I think that’s where ultimately all of this could go is that integrated experience.
(30:25):
I have no idea how we get there, but okay. Data mesh, it’s a schema. The way people describe the data mesh is decentralized data ownership. So, it is rather than having data be centralized into a single team, and that team distributed out to everybody else.
(30:48):
It’s individual teams own their component parts of it in alignment with the way that the centralized team would say these are best practices. And then, that way, the people who own the data as it is produced also own the output of it and things like that.
(31:06):
So, it’s less like funnel it through a middleman. It’s more of, okay, you are the marketing team, this is your component of the data mesh that you own. And so, there is more decentralized ownership. I guess, it seems hard to manage and practice.
(31:22):
The way I’ve seen people describe it is basically it’s the thing that you naturally create when you’re a very big organization and you can’t have a centralized data team that can possibly centralize everything, which is fair but uninteresting, I guess, but I don’t know.
(31:39):
This is one of those that I’ve… the only way I can understand it is something that seems simpler than it should be. And once it gets more complicated, I am no longer smart enough to understand it.
Matt Turck (31:53):
What’s a bull case for this whole space and reasons to be excited about the next few years, trends or what have you?
Benn Stancil (32:15):
To me, it is things like these data products basically, where if that is the way that everything gets done and the expectation is that is the way everything gets done, then what the data landscape becomes is a second version of cloud infrastructure essentially.
(32:33):
Where if we are building products on top of… if data is the core thing that we need to build products on top of, you start to have to build an entire collection of services and stuff around it to support that. I don’t know if it’s as big as web hosting stuff.
(32:47):
But it becomes something where like Snowflake’s ambition to me. Snowflake’s ambition is as best I can parse it, not just to be a database, but to be this platform on which you can build things. And so, if I want, I could run an entire company on top of Snowflake.
(33:05):
If you can do that, then you start to say, okay, there’s a bunch of technology underneath this that being able to do these enables like being able to build a product from top of Snowflake enables me to do where I can build all of these integrated services into my product.
(33:18):
Again, the Figma example or ways that people do marketing now with a lot of automated marketing tooling. All that stuff can be rebuilt on top of a data infrastructure instead of on top of just AWS and S3 and EC2 and all that stuff. So, I think the thing that the ecosystem gets really big is that.
(33:40):
Is that there becomes of entire developers on top of it that isn’t just people building tools for data companies, but are people building products that are fundamentally unseparable from the modern data stack or whatever that collection of things is.
(33:59):
That’s how you get really big. Beyond that, it’s more like data teams become popular and so everybody just needs a bunch of data products. And that seems like the median outcome is the data philosophies of Facebook and LinkedIn and all these early tech companies gets adopted by the enterprise.
(34:17):
And so, all of these modern data tools that tech companies buy today go off and get sold to Coca-Cola and Caterpillar and all that stuff. And that market’s big. It’s not that big, it’s not enough to support a thousand unicorns, but it’s big.
Matt Turck (34:33):
And these are a path or a world where what seems to be this constant reinvention of tools to solve the same problem. Does that stop? I’m referring to there was the whole wave for Hadoop and then cloud vendors at some point, like everybody was saying, “Well, cloud is going to solve it all.”
(34:54):
And then, that evolve to Snowflake puts Kubernetes and that evolve into the modern data stack. Does it ever stop? Or, every five years, we’re just going to collectively reinvent the whole thing?
Benn Stancil (35:05):
Probably not. I mean, there’s-
Matt Turck (35:06):
Good for my business.
Benn Stancil (35:10):
Yeah. VC speaking to Ponzi schemes. No. And I think a lot of it is because there’s a pendulum that swings back and forth on this stuff, where this whole… is airflow being unbundled or rebundled or bundled in a different, the conversation six months ago.
(35:29):
That type of conversation of unbundling tools and then rebundling them, I think, we’ll go back and forth on that forever, where take the Snowflake piece. Snowflake becomes a database, then they become this data platform. We all love all the features.
(35:45):
But then, Firebolt comes along and says, “No, we’re just the super-fast database.” We’re like, “Oh, a database without all the features.” Great, that’s way better. And then, Firebolt becomes popular. And then, we’re like, “Wait, but maybe if we tack on all these features, that’ll be really great too.”
(35:58):
And so, I think there is that pendulum that I think will happen inevitably where there will always be some, oh, we’ve specialized too much, let’s make a generalized tool. We have a generalized tool, let’s specialize. Does that represent real steps forward? I don’t know, probably in some ways.
(36:17):
But I think there’s like we’ll always be enough. The space has gotten big enough now. I think we have somewhat of a perpetual emotion machine of reinvention at this point.
Matt Turck (36:27):
Great. I want to open up four questions in a minute, but maybe too close. Let’s actually talk about Mode. What does Mode do today? What’s the roadmap? What are you excited about?
Benn Stancil (36:45):
So, Mode is a BI analytics product. It sits on top of your warehouse. It has a sequel ID, has a visualization tool similar to something like you get in Tableau. Has some embedded notebooks. The idea behind it is basically data teams have to provide reporting to businesses, that is a core part of their function.
(37:04):
They have traditionally not liked the way they’ve had to do it. They don’t want LookML and Looker is great. But a lot of analysts aren’t wanting to write LookML all day. They want to do tool… use tools that are more native to them, but you still have to provide the dashboarding experience.
(37:18):
And so, our view is how do we get it so that… how do we build a tool that can solve the BI and self-serve reporting problem while also doing it in a way that is more comfortable for analysts and is comfortable for their end users as well. And so, for us, it’s about bringing those experiences together.
(37:33):
We don’t see it as reinventing notebooks or reinventing visualizations. It’s more of what are the best experiences that we can provide to people in those different form function… form factors and then give them all in one seamless way. So, what does that mean for the roadmap?
(37:48):
It’s largely about how do we think about bringing those tools together and bringing the people who are working on them together in better ways. The other place where we see pushing the roadmap is our view is the data stack is basically turned on its side where it used to be BI tools would be governance. They would be visualization. They would sometimes be storage.
(38:10):
Those things have since been separated out where storage is its own layer. Governance and transformation are its own layer, and we see consumption is its own layer. So, instead of building a BI tool that is integrated with its own data modeling layer, we see it as how do we integrate with the data modeling layers people want to use like dbt.
(38:28):
If they’re wanting to use some of the newer stuff like Transform for instance, that they’ve pivoted to some degree. But the other tools there are ways to do semantics in the database rather than that living in your BI tool. We think that should live in a more generalized layer and then we just consume from it.
Matt Turck (38:43):
Very good. All right. As promised, I want to open to questions if there are some. All right. I’ll [inaudible 00:38:52] his in first. You’ll be next.
Speaker 3 (38:56):
Anyway, interesting talk. I don’t know where to start. But I’m just going to seize on one point that you were making, which you were talking about how problems have gotten so fragmented, there were so… well, that’s a point problem, so you were given like dbt and FiveTran as examples.
(39:12):
What I’m wondering is, is the end state that you’re looking for a declarative approach where you say, like in Star Trek, hey, data pipeline, I want to have this information by 8:00 so I can answer this question at that point. Question I have here. It’s two-halves, the question.
(39:29):
One, has the industry, has the landscape, the industry landscape, the vendor landscape, technology landscape gotten too fragmented to make that happen? And second half of the question is, the answer to that, solution to that being more vertical integration? I know Snowflake acquires upstream data breaks, acquires upstream, et cetera, etcetera.
Benn Stancil (39:50):
So, yes, it probably has gotten too fragmented for that to be like well done today. That’s the challenge I would pose to folks at Astronomer of how do you solve this problem. The one way is potentially get verticalized again. So, Snowflake starts a database.
(40:09):
Now, they start building up the stack and say, “Great, we can integrate with all these things because we just provide those services.” This also, to me, is the more likely model is something like the way that cloud providers work where they are separate products that can technically work across different products but you largely just buy them from one service because they’re neatly coupled.
(40:29):
So, again, I can integrate a bunch of AWS services together really easily, but they’re separate products. Outside of that, I don’t actually know how you… the… it’s a very difficult thing to get a bunch of these tools to talk the same language. I think there are ways to get there.
(40:49):
I don’t think the way we get there is through open standards and stuff like that. I don’t think anybody will actually adhere to that. I think most likely what happens is Snowflake basically says, “Hey, if you do things in this particular way, we can integrate with you.”
(41:02):
And then, a bunch of people are like, well, there’s a lot of gravity around Snowflake, we’ll build into that piece, that becomes the dominant standard. dbt is actually doing a little bit as already. They don’t quite have the APIs into it, the way that you might want.
(41:15):
But a lot of people are starting to circle around dbt standards as a way to think about this stuff. There’s a lot of gentrification now of things that are happening in the data world because dbt has made that a concept people understand. So, I could see that happening where it’s… we find some pole that we all gravitate around, but it’s still too fragmented for that to be that realistic at this point.
Speaker 4 (41:43):
This is a similar question. I mean, going to Data Council, I saw that is a smaller event than something like an RSA in security and potentially a larger market. So, maybe three to five years out, do you see less players in the data space? And is that driven by consolidation going to some of those cloud providers or just because you think the space is overvalued and maybe Matt can’t sleep tonight because he got a lot of capital deployed.
Benn Stancil (42:13):
Probably, are less companies in the space. I think it’s less that there’s less companies. It’s more that today in a place like Data Council, which again, I have no, nothing bad to say about the conference, there’s a lot of startups and roughly the same face.
(42:32):
There’s a lot of startups between A to series A to series C that have raised somewhere between $10 and a $100 million, which is a round in 2019 or 2020. I don’t think we have that where there’s a bunch of companies that are all chasing very big outcomes, where there aren’t clear winners yet.
(42:52):
I think there will be more this is the winner in this particular part of the ecosystem. There’s a lot of smaller players trying to figure out where do they fit in. But now, it feels like everybody is still chasing the very big outcome. Another way I put this is, we’re still in a phase where it feels like the platforms haven’t yet been defined.
(43:12):
Where everybody wants to be the Apple app store, not many of us are going to actually be. And at some point, we just got to chase building the apps that are going to make not enormous amounts of money, but will make enough to make a sustainable business.
(43:25):
I think because nothing is settled yet, a lot of people are chasing like can I be the canonical platform in this space? And so, you have much bigger ambitions there than everybody can achieve. It doesn’t mean some people won’t, but everybody wants to be the standard for their particular piece of the industry because it’s still a free for able to do that.
(43:43):
And I don’t think that is still the case. I don’t think it’s the standard… right now, the only standards are like there’s a handful of databases. dbt somehow still operates in a space that has essentially no competition, which I don’t know how they pulled that off.
(43:54):
But outside of that, there’s not really, I mean, even like BI, which is a pretty established corner of the market, there’s not a standard. There’s not like the thing that everybody goes out and buys. And so, I think there’ll be more of that by that point.
(44:06):
And so, it’s more of figuring out the corners to operate and instead of who’s going to be the standard observability tool, the standard ETL tool, the standard… are those things even need… the things that need standards. I think that’ll be more settled.
Matt Turck (44:17):
All right, cool. Last one.
Speaker 5 (44:19):
Hi. Because of the lack of standards that you mentioned, do you think that there is a scope for proprietary databases like something that’s being specific in the startup world that one could actually just cater if you have the human resource and the brain power to write proprietary databases, rather than relying on something like Snowflake or anything that’s out there? Have you come across any such proprietary databases in your-
Benn Stancil (44:48):
Snowflake is a proprietary database, but proprietary in the sense that?
Speaker 5 (44:51):
Meaning something that domains specific, if I want to startup.
Benn Stancil (44:55):
So, a database for-
Speaker 5 (44:56):
Yeah, just for-
Benn Stancil (44:57):
… climate stuff, I don’t know. I’m making this up. Yeah. I mean, I would think that there would be… this, I guess, it gets actually a little bit to your question, which is, yeah, we’re like that’s probably what happens. Is at some point, you stop chasing, can we be the next cloud data warehouse?
(45:18):
I mean, everybody will always be chasing that a little bit. There will always be someone who’s like going to disrupt Snowflake in the same way. Oracle didn’t win forever and Microsoft didn’t win forever. But that becomes a much harder sell. And probably what you end up chasing is where are the places where Snowflake really struggles?
(45:33):
Graph databases, maybe Snowflake really struggles in places where that’s useful. Or for particular verticals, as you said. Maybe there’s stuff in finance, I don’t know. Crypto might have special databases type of… I have no idea how crypto works, but maybe there’s stuff, particular things there that work really well. So, I could see that. But that is a little bit of the moons orbiting the planet rather than everybody trying to be the planet.
Matt Turck (45:57):
Great. Well, that feels like a wonderful place to leave it. Thank you so much. This was terrific. Really enjoyed it. Thanks for coming back. And I hope you’ll come back again.
Benn Stancil (46:04):
Thank you.