Like many in the NYC tech community, I was excited to hear the news last Friday night that data startup Hyperpublic was acquired by Groupon.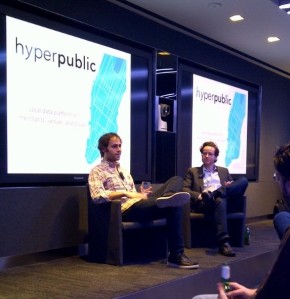
In addition to the fact that he was the first speaker we ever had at our NYC Data Business Meetup (which should put him in a very special place in history, right there… right?), Jordan Cooper, Hyperpublic’s co-founder and CEO, is a terrific entrepreneur, very thoughtful about the data space, and well liked and respected by all who know him in the tech community. He and his co-founder Doug Petkanics built an awesome team (including scoring a big win when they recruited Jeff Weinstein), got some great seed investors (including Lerer Ventures and Thrive Capital, which also had another quick win with GroupMe a few months ago), and it’s always nice to see good things happening to good people.
This is also good news for the budding big data and enterprise tech community in NYC. Hyperpublic was very much part of an emerging ecosystem of interesting, tech heavy companies in NYC, that includes 10gen (MongoDB), Enterpoid, Nodejitsu, Datadog, Opani, Mortar Data, etc., and seeing a young company successfully go through the cycle of product creation, seed funding and exit is encouraging for aspiring entrepreneurs in that space.
Hyperpublic was tackling some difficult engineering problems. As a geo-local data company, they pulled data from many different sources (through both APIs and web crawling) and then organized and structured it in a way that developers could use to build location based applications. The difficulty of this type of effort comes from ensuring that the data has enough (i) breadth (defined by the number of geographic regions where they have a critical mass of relevant data – they got to 50 cities), (ii) depth (how detailed the data about each point of interest is) and (iii) freshness (for example being able to pick up on location openings and closings). All of this requires an ability to gather, normalize and cross-reference enormous amounts of unstructured data at rapid intervals, and doing it with high levels of certainty is not a simple task.
Hyperpublic made very solid inroads in terms of addressing those challenges, due in large part to the quality of the engineering team and culture that Jordan and Doug had managed to put together. Both from a technology and team perspective, the acquisition makes perfect sense for Groupon, as they ramp up their effort in the mobile local space.
From an industry watch perspective, however, the acquisition highlights the difficulty of building long term, standalone businesses based purely on gathering data and making it available to developers, including in the geo local space. Simple Geo tried this as well and ended up being acquired by Urban Airship for $3.5 million. Urban Airship announced quickly afterwards that it was shutting down Simple Geo services altogether, leaving developers that were relying on it pretty much stranded. Hyperpublic presumably fared a lot better, but Groupon acquired it for its own use (as a needed element to its infrastructure), and will also stop providing the data to developers, effective March 2. It will be interesting to see how companies like Factual (which covers more than geo-local data) and PlaceIQ (which has a slightly different twist on geo-local) evolve in that context.
Startups that are in the business of gathering data and providing it to developers (as opposed to selling it to the enterprise) can get rapid user adoption, but typically face a revenue model challenge. In addition, particularly in the geo local data space, there is an uncertainty around how to work in the long term with the larger companies that capture a lot of the geo local data, as a by product of their core activity. Are they friends or foes? Many of the large consumer internet companies have a geo local API (Facebook Graph API, Foursquare Venue Database, Google Places API, Microsoft Bing Maps Location API, Yahoo Local API, Yelp API), which the data companies have used as one of their sources. While those large internet consumer companies have been happy so far to cooperate with the data companies, how long will they continue to do so, if it turns out that the data becomes a real potential source of revenue?
From Hyperpublic’s perspective, therefore, the timing of the acquisition makes perfect sense – they built the team and the technology, and took the company to the stage where a natural acquirer like Groupon could come in and acquire them. Taking it to the next level, while certainly possible, would have required a significant amount of additional time and money, and possibly figuring out a consumer facing product to start capturing their own geo local data, in a context where uncertainty about the revenue model and the competition could have made things tricky.
As a side note, it is exciting to see recently-IPO’ed Groupon getting into acquisition mode quickly (see also Kima Labs), and one can only get excited imagining how acquisitive Facebook is going to be after it goes public.
Congrats to Jordan, Doug, Jeff and the Hyperpublic team!